Tutorial Materials

This book is still in development. Everything is currently subject to change.
Welcome the materials on past demos, workshops and tutorials hosted for the Brane framework!
This book represents auxillary material for live sessions. Most likely, you are looking for the User Guide or the Specification. However, the resources in this book may still be useful as additional material on understanding Brane.
You can check the next chapter for an overview of all the materials hosted on this site. Alternatively, check the sidebar on the left for general navigation.
Attribution
The icons used in this book (,
,
) are provided by Flaticon.
Overview
This chapter lists all the materials hosted in this book.
We make a subdivision of the contents based on purpose:
- The tutorials-series hosts materials used in tutorials and demos about Brane.
See below for an overview of the classes or sessions per-type.
Tutorials
Various tutorials/demos have been given about Brane:
- (2023-04-20) At ICT.OPEN 2023
- (2023-10-23) At UMC Utrecht, as part of concluding the EPI Project
Next
Click on any of the links above to navigate to the series or chapter that details the material you want to look at. Or select something from the sidebar on the left.
Tutorials
In this chapter, you can find the resources used in tutorials hosted to promote the BRANE framework.
The resources are ordered by date of tutorial. The first tutorial will be hosted at ICT.OPEN, 20-04-2023.
In addition, the most recent resources to host a tutorial are:
- The presentation (from the tutorial on 20-04-2023)
- The handout (from the tutorial on 20-04-2023)
- The package tutorial (from the tutorial on 20-04-2023)
- The workflow tutorial (from the tutorial on 20-04-2023)
Overview
An overview of all tutorials, ordered chronologically:
You can also select a tutorial in the sidebar to the left.
First BRANE tutorial at ICT.OPEN
The first tutorial that introduced users to the BRANE framework is given at ICT.OPEN 2023, a conference aiming to bring academia and industry together. Its purpose is to have users experience the role as a software engineer and scientist in the framework, mainly to develop an understanding of how working with the framework looks like in practise.
The tutorial is written for framework version 2.0.0.
The tutorial consists of the following parts:
- 12:30-12:45: Introduction (presentation)
- 12:45-13:30: Part1: Hello, world! (guided hands-on)
- 13:30-13:45: Break
- 13:45-14:15: Part 2: A workflow for Disaster Tweets (hands-on)
- 14:15-14:30: Evaluation
The following resources are used, which are hosted on this website:
- Generic handout (here)
- Handout for Part 1: Hello, world! (here)
- Handout for Part 2: A workflow for disaster tweets (here)
- Introduction slides (here)
Part 1: Hello, world!
In this document, we will detail the steps to take to write a simple package within the EPI Framework (also called the BRANE Framework). Specifically, this tutorial will focus on how to install the CLI client (the brane
executable), build a hello_world
package and then call the hello_world()
function within that package on a local machine.
Background
The framework revolves around workflows, which are high-level descriptions of an algorithm or data processing pipeline that the system will execute. Specifically, every workflow contains zero or more tasks, which are conceptual functions that take an in- and output, composed in a particular order or control flow. It helps to think of them as graphs, where the nodes are tasks that must be called, and the edges are some form of data flowing between them. An example of such a workflow is given in Figure 1.
Figure 1: A very simple workflow using three tasks, f
, g
and h
. The nodes represent a function call, whereas the edges represent some data dependency. Specifically, this workflow depicts f
has to be run first, then a second call of f
and a new call of h
can run in parallel, after which a third function g
must be called.
While workflows can be expressed in any kind of language, the EPI Framework features its own Domain-Specific Language (DSL) to do so, called BraneScript1. This language is very script-like, which allows us to think of tasks as a special kind of function. Any control flow (i.e., dependencies between tasks) is then given using variables and commonly used structures, such as if-statements, for-loops, while-loops, and less commonly used structures, such as on-structs or parallel-statements.
Objective
In this tutorial, we will mainly focus on creating a single task to execute. Traditionally, this task will be called hello_world()
, and will return a string "Hello, world!"
once it is called. To illustrate, the following Python snippet implements the logic of the task:
def hello_world():
return "Hello, world!"
By using print(hello_world())
, we can print Hello, world!
to the terminal. In the EPI Framework, we will implement the function as a task, and then write a very simple workflow that implements the print-part.
There is a second DSL, Bakery, which is more unique to the framework and features a natural language-like syntax. However, this language is still in development, and so we focus on BraneScript.
Installation
To start, first download the brane
executable from the repository. This is a command line-based client for the framework, providinh a wide range of tools to use to develop packages and workflows for the EPI Framework. We will use it to build and then test a package, which can contain one or more tasks. Since we are only creating the hello_world()
task, our package (called hello_world
) will contain only one task.
The executable is pre-compiled for Windows, macOS (Intel and M1/M2) and Linux. The binaries in the repository follow the pattern of <name>-<os>-<arch>
, where <name>
is the name of the executable (brane
for us), <os>
is an identifier representing the target OS (windows
for Windows, darwin
for macOS and linux
for Linux), and <arch>
is the target processor architecture (x86_64
, typically, or aarch64
for M1/M2 Macs).
So, for example, download brane-windows-x86_64
if you are on Windows, or brane-darwin-aarch64
if you have an M1/M2 Mac. You can see the commands below for the most likely executable per OS/architecture.
When in doubt, choose
x86_64
for your processor architecture. Or ask a tutorial host.
Once downloaded, it is recommended to rename the executable to brane
to follow the calling convention we are using in the remainder of this document. Open a terminal in the folder where you downloaded the executable (probably Downloads
), and run:
:: For Windows
move .\brane-windows-x86_64 .\brane
# For macOS (Intel)
mv ./brane-darwin-x86_64 ./brane
# For macOS (M1/M2)
mv ./brane-darwin-aarch64 ./brane
# For Linux
mv ./brane-linux-x86_64 ./brane
If you are on Unix, you probably want to execute a second step: by just renaming the executable, you would have to call it using ./brane
instead of brane
. To fix that, add the executable to somewhere in your PATH, e.g.:
sudo mv ./brane /usr/local/bin/brane
If you installed it successfully, you can then run:
brane --version
without getting brane not found
errors.
If you don't want to put
brane
in your PATH, you can also replace all occurrences ofbrane
with./brane
in the subsequent commands (or any other path/name). Additionally, you can also run:export PATH="$PATH:$(pwd)"
to add your current directory to the PATH variable. However, note that this lasts only for your current terminal window, and until you restart it.
Writing the code
The next step is to write the code that we will be running when we execute the task. In the EPI Framework, tasks are bundled in packages; and every package is implemented in a container. This means that every task has its own dependencies shipped within, and that multiple tasks can share the same dependencies. This also means that a task can be implemented in any language, as long as the program follows a particular convention in how it takes input and writes output. Specifically, the EPI Framework will call a specific executable file with a specific set of arguments and environment variables set, and then receive return values from it by reading the executable's stdout
.
For the purpose of this tutorial, though, will choose Python to implement our hello_world
-function. Because our function is so simple, we will only need a single file, which we will call hello.py
. Create it, and then write the following in it (including comments):
#!/usr/bin/env python3
def hello_world():
return "Hello, world!"
print(f'output: "{hello_world()}"')
Let's break this down:
- The first line,
#!/usr/bin/env python3
is a line that tells the operating system that this file is a Python script (it defines it must be called with thepython3
executable). Any file that has this on the first line can be called by just calling the file instead of having to prefixpython3
, e.g.,
instead of./hello.py
This is important, because the framework will use the first calling convention.python3 hello.py
- The function,
def hello_world(): ...
, is the same function as presented before; it simply returns the"Hello, world!"
string. This is actually the functionality we want to implement. - The final line,
print(f'output: "{hello_world()}"')
prints the generated string to stdout. Note, however, that we wrap the value in quotes ("
) and prefix it withoutput:
we do this because of the convention that packages for the EPI Framework have to follow. The framework expects the output to be given in the YAML format, under a specific name. We chooseoutput
(see below).
And that's it! You can save and close the file, while we will move to the second part of a package: the container.yml
file.
Writing the container.yml
A few text files do not make a package. In addition to the raw code, the EPI Framework also needs to know some metadata of a package. This includes things such as its name, its version and its owners, but, more importantly, also which tasks the package contributes.
This information is conventionally contributed using a file called container.yml
. This is another YAML file where the toplevel keys contribute various pieces of metadata. Create a file with that name, and then write the following to it:
# A few generic properties of the file
name: hello_world
version: 1.0.0
kind: ecu
# Defines things we need to install
dependencies:
- python3
# Specifies the files we need to put in this package
files:
- hello.py
# Defines which of the files is the file that the framework will call
entrypoint:
kind: task
exec: hello.py
# Defines the tasks in this package
actions:
'hello_world':
command:
input:
output:
- name: output
type: string
This is quite a lot, so we will break it down in the following subsections. Every subsection will contain the highlighted part of the container.yml
first, and then uses three dots (...
) to indicate parts that have been left out for that snippet.
Minimal metadata
# A few generic properties of the file
name: hello_world
version: 1.0.0
kind: ecu
...
The top of the file starts by providing the bare minimum information that the EPI Framework has to know. First are the name of the package (name
) and the version number (version
). Together, they form the identifier of the package, which is how the system knows which package we are calling tasks from.
Then there is also the kind
-field, which determines what kind of package this is. Currently, the only fully implemented package kind is an Executable Code Unit (ECU), which is a collection of arbitrary code files. However, other packages types that will be supported in the future are OpenAPI-packages and packages BraneScript or Bakery.
Specifying dependencies
...
# Defines things we need to install
dependencies:
- python3
...
Because packages are implemented as containers, we have the freedom to specify the set of dependencies to install in the container. By default, the framework uses Ubuntu 20.04
as its base image, and the dependencies specified are apt-packages. Note that the base container is fairly minimal, and so we have to specify we need Python installed (which is distributed as the python3
-package).
Collecting files
...
# Specifies the files we need to put in this package
files:
- hello.py
...
Then the framework also has to know which files to put in the package. Because we have only one file, this is relatively simply: just the hello.py
file. Note that any filepath is, by default, relative to the container.yml
file itself; so by just writing hello.py
we mean that the framework needs to include a file with that name in the same folder as container.yml
.
The files included will, by default, mimic the file structure that is defined. So if you include a file that is in some directory, then it will also be in that directory in the resulting package. For example, if you include:
files: - foo/hello.py
then it will be put in a
foo
directory in the container as well.
Setting the entrypoint
...
# Defines which of the files is the file that the framework will call
entrypoint:
kind: task
exec: hello.py
...
Large projects typically have multiple files, and only one of them serves as the entrypoint for that project. Moreover, not every file included will be executable code; and thus it is relevant for the framework to know which file it must call. This is specified in this snippet: we define that the hello.py
file in the container's root is the one to call first.
As already mentioned, the framework will call the executably "directly" (e.g., ./hello.py
in this case). This means that, if the file is a script (like ours), we need a shebang (e.g., #!/usr/bin/env python3
) string to tell the OS how to call it.
Even if your package implements multiple tasks, it can only have a single entrypoint. To this end, most packages define a simple entrypoint script that takes the input arguments and uses that to call an appropriate second script or executable for the task at hand.
Defining tasks
...
# Defines the tasks in this package
actions:
hello_world:
command:
input:
output:
- name: output
type: string
The final part of the YAML-file specifies the most important part: which tasks can be found in your container, and how the framework can call them.
In our container, we only have a single task (hello_world
), and so we only have one entry. Then, if required, we can define a command-line argument to pass to the entrypoint to distinguish between tasks (the command
-field). In our case, this is not necessary because we only have a single one, and so it is empty.
Next, one can specify inputs to the specific task. These are like function arguments, and are defined by a name and a specific data type. At runtime, the framework will serialize the value to JSON and make these available to the entrypoint using environment variables. However, because our hello_world()
function does not need any, we can leave the input
-field empty too.
Finally, in the output
section, we can define any return value our task has. Similar to the input, it is defined by a name
and a type
. The name given must match the name returned by the executable. Specifically, we returned output: ...
in our Python script, meaning that we must name the output variable output
here as well. Then, because the output itself is a string, we denote it as such by using the type: string
.
In summary, the above actions
field defines a single function that has the following pseudo-signature:
hello_world() -> string
Building a package
After you have a container.yml
file and the matching code (hello.py
), it is time to build the package. We will use the brane
CLI-tool for this, and requires Docker and the Docker Buildx-plugin to be installed.
On Windows and macOS, you should install Docker Desktop, which already includes the Buildx-plugin. On Linux, install the Docker engine for your distro (Debian, Ubuntu, Arch Linux), and then install the Buildx plugin using:
# Install the executable
docker buildx bake "https://github.com/docker/buildx.git"
mkdir -p ~/.docker/cli-plugins
mv ./bin/build/buildx ~/.docker/cli-plugins/docker-buildx
# Create a build container to use
docker buildx create --use
If you have everything installed, you can then build the package container using:
brane build ./container.yml
The executable will work for a bit, and should eventually let you know its done with:

If you then run
brane list
you should see your hello_world
container there. Congratulations!
Running your package
All that remains is to see it in action! The brane
executable has multiple ways of running packages locally: running tasks in isolation in a test-environment, or by running a local workflow. We will do both of these in this section.
The test environment
The brane test
-subcommand implements a suite for testing single tasks in a package, in isolation. If you run it for a specific package, you can use a simple terminal interface to select the task to run, define its input and witness its output. In our case, we can call it with:
brane test hello_world
This should show you something like:

If you hit Enter, the tool will query you for input parameters - but since there are none, instead it will proceed to execution immediately. If you wait a bit, you will eventually see:

And that's indeed the string we want to see!
The first time you run a newly built package, you will likely see some additional delay when executing it. This is because the Docker backend has to load the container first. However, if you re-run the same task, you should see a significant speedup compared to the first time because the container has been cached.
Running a local workflow
The above is, however, not very interesting. We can verify the function works, but we cannot do anything with its result.
Instead of using the test environment, we can also write a very simple workflow with only one task. To do so, create a new file called workflow.bs
, and write the following in it:
import hello_world;
println(hello_world());
Let's examine what happens in this workflow:
- In the first line,
import hello_world;
, we tell the framework which package to use. We reference our package by its name, and because we omit a specific version, we let the framework pick the latest version for us. - In the second line,
println(hello_world());
, we call ourhello_world()
task. The result of it will be passed to a builtin function,println()
, which will print it to the stdout.
Save the file, close the editor, and then run the following in your terminal to run the workflow:
brane run ./workflow.bs
If everything is alright, you should see:

The
brane
-tool also features an interactive Read-Eval-Print Loop (REPL) that you can use to write workflows as well. Runbrane repl
, and then you can write the two lines of your workflow separately:![]()
Because it is interactive, you can be more flexible and call it repeatedly, for example:
![]()
Simply type
exit
to quit the REPL.
Conclusion
And that's it! You've successfully written your first EPI Framework package, and then you ran it locally and verified it worked.
In the second half of the tutorial, we will focus more on workflows, and write one for an extensive package already developed by students. You can find the matching handout here.
Part 2: A workflow for Disaster Tweets
In this document, we detail the steps that can be taken during the second part of the tutorial. In this part, participants will write a larger workflow file for an already existing package and submit it to a running EPI Framework instance. The package implements a data pipeline for doing Natural Language Processing (NLP) on the Disaster Tweets dataset, created for the matching Kaggle challenge.
Background
In the first part of the tutorial, you've created your own Hello, world!-package. In this tutorial, we will assume a more complex package has already been created, and you will take on the role as a Domain-Specific Scientist who wants to use it in the framework.
The pipeline implements a classifier that aims to predict is a tweet is indicating a natural disaster is happening, or not. To do so, a naive bayes classifier has been implemented that takes preprocessed tweets as input, and outputs a 1
if it references a disaster, or a 0
if it does not. In addition, various visualisations have been implemented that can be used to analyse the model and the dataset.
The package has been implemented by Andrea Marino and Jingye Wang for the course Web Services and Cloud-Based Systems. Their original code can be found here, but we will be working with a version compatible with the most recent version of the framework which can be found here.
Objective
As already mentioned, this part focusses on implementing a workflow that can do classification on the disaster tweets dataset. To do so, the dataset has to be downloaded and the two packages have to be built. Then, a workflow should be written that does the following:
- Clean the training and test datasets (
clean()
) - Tokenize the training and test datasets (
tokenize()
) - Remove stopwords from the tweets in both datasets (
remove_stopwords()
) - Vectorize the datasets (
create_vectors()
) - Train the model (
train_model()
)
All of these functions can be found in the compute
package.
Then, optionally, any number of visualizations can be implemented as well to obtain results from the dataset and the model. Conveniently, you can generate all of them in a convenient HTML file by calling the visualization_action()
function from the visualization
package, but you can also generate the plots separately.
Tip: If you use
brane inspect <package>
, you can see the tasks defined in a package together with which input and output the tasks define. For example:![]()
Installation
Before you can begin writing your workflow, you should first built the packages and download the required datasets. We will treat both of these separately in this section.
We assume that you have already completed part 1. If not, install the brane
executable and install Docker as specified in the previous document before you continue.
Building packages
Because the package is in a GitHub repository, this step is actually fairly easy by using the brane import
command.
Open a terminal that has access to the brane
-command, and then run:
brane import epi-project/brane-disaster-tweets-example -c packages/compute/container.yml
brane import epi-project/brane-disaster-tweets-example -c packages/visualization/container.yml
This will allow you to build a package's source from a repository of the user epi-project
and that goes by the name of brane-disaster-tweets-example
. An eagle-eyed person may notice that this is exactly the URL of a repository, except that https://github.com/
is omitted. The second part of the command, -c ...
, specifies which container.yml
to use in that repository. We need to specify this because the repository defines two different packages, but this does allow us to build both of them.
After the command completes, you can verify that you have them installed by running brane list
again.
Obtaining data
In the EPI Framework, datasets are considered assets, much like packages. That means that similarly, we will have to get the data file(s), defined some metadata, and then use the brane
tool to build the assets and make them available for local execution.
To save some time, we have already pre-packaged the training dataset here, and the test dataset here. These are both ZIP-archives containing a directory with a metadata file (data.yml
) and another directory with the data in it (data/dataset.csv
). Once downloaded, you should unpack them, and then open a terminal.
Navigate to the folder of the training dataset first, and then run this command:
brane data build ./data.yml
Once it completes, navigate to the directory of the second dataset and repeat the command. You can then use brane data list
to assert they have been added successfully.
The data.yml
file itself is relatively straightforward, and so we encourage you to take a look at it yourself. Similarly, also take a look at the dataset itself to see what the pipeline will be working on.
By default, the above command does not copy the dataset file referenced in
data.yml
, but instead just links it. This is usually fine, but if you intend to delete the downloaded files immediately afterwards, use the--no-links
flag to copy the dataset instead.
Writing the workflow - Compute
Once you have prepared your local machine for the package and the data, it is time to write a proper workflow!
To do so, open a new file (called, for example, workflow.bs
) in which we will write the workflow. Then, let's start by including the packages we need:
import compute;
import visualization;
The first package implements everything up to training the classifier, and the visualization package implements functions that generate graphs to inspect the dataset and the model, so we'll need both of them to see anything useful.
Next up, we'll do something new:
// ... imports
// We refer to the datasets we want to use
let train := new Data{ name := "nlp_train" };
let test := new Data{ name := "nlp_test" };
The first step is to decide which data we want to use in this pipeline. This is done by creating an instance of the builtin Data
class, which we can give a name to refer to a dataset in the instance. If you check brane data list
, you'll see that nlp_train
is the identifier of the training set, and nlp_test
is the identifier of the test set.
Note, however, that this is merely a data reference. The variable does not represent the data itself, and cannot be inspected from within BraneScript (you may not that the Data
class has no functions, for example). Instead, its only job is so that the framework knows which dataset to attach to which task at which moment. You can verify that the framework attaches it by inspecting the package code and observing that it will pass the task a path where it can find the dataset in question.
Next, we will do the first step: cleanup the dataset.
// ... datasets
// Preprocess the datasets
let train_clean := clean(train);
let test_clean := clean(test);
You can see that this is the same function that takes different datasets as input, and then returns a new dataset that contains the same data, but cleaned. Note, however, that this dataset won't be externally reachable; instead, we call it an intermediate result, which is a dataset which will be deleted after the workflow completes.
Let's continue, and tokenize and then remove stopwords from the two datasets:
// ... cleaning
let train_final := tokenize(train_clean);
let test_final := tokenize(test_clean);
train_final := remove_stopwords(train_final);
test_final := remove_stopwords(test_final);
As you can see, we don't need a new variable for every new result; we can just override old ones if we don't need them anymore.
Now that we have preprocessed datasets, we will vectorize them so that it becomes quicker for a subsequent call to load them. However, by design of the package, these datasets are vectorized together; so we have to give them both as input, and only get a single result containing both output files:
// ... preprocessing
let vectors := create_vectors(train_final, test_final);
And with that, we have a fully preprocessed dataset. That means we can now train the classifier, which is done conveniently by calling a single function:
// ... vectorization
let model := train_model(train, vectors);
commit_result("nlp_model", model);
The second line is the most interesting here, because we are using the builtin commit_result
-function to "promote" the result of the function to a publicly available dataset. Specifically, we tell the framework to make the intermediate result in the model
-variable public under the identifier nlp_model
. By doing this, we can later write a workflow that simply references that model in the first place, and pickup where we left off.
You might notice that the model is returned as a dataset as well. While the function could have returned a class or array in BraneScript to represent it, this has two disadvantages:
- Most Python libraries write models to files anyway, so converting them to BraneScript values needs additional work; and
- By making something a dataset, it becomes subject to policy. This means that participating domains will be able to say something about where the result may go. For this reason, in practice, a package will likely not be approved by a hospital if it does not return important values like these as a dataset so that they can stay in control of it.
This is useful to remember if you ever find yourself writing BraneScript packages again.
And with that, we have a workflow that can train a binary classifier on the Disaster Tweets dataset! However, we are not doing anything with the classifier yet; that will be done in the next section.
Writing a workflow - Visualization
The next step is to add inference to the network, and to generate some plots that can show it works. To do so, we will add a few extra function calls at the bottom of your workflow.bs
file.
You can also easily create a new workflow file to separate training and inference. If you want to, create a new workflow file and try to write the start yourself. You will probably have to commit the cleaned and final datasets in the previous workflow, and then use them and the model here. Also, don't forget to add the
import
s on top of your file.
Scroll past the training, and write the following:
// ... training
// Create a "submission", i.e., classify the test set
let submission := create_submission(test, vectors, model);
This line will use the existing test set, its vectors (the training-vectors are unused) and the trained model to create a so-called submission. This is just a small dataset that matches tweet identifiers to the prediction the model made (1
if it classified it as a disaster tweet, or 0
otherwise). The terminology stems from the package being written for a Kaggle challenge, where this classification has to be submitted to achieve a particular score.
We can then use this submission to generate the visualizations. The easiest way is to call the visualization_action()
function from the visualization
package:
// ... submission
// Create the plots, bundled in an HTML file
let plot := visualization_action(
train,
test,
submission
);
return commit_result("nlp_plot", plot);
Here, we call the function (which takes both datasets and the classification), and commit its resulting plot. Note, however, that we return
this dataset from the workflow. This means that, upon completion, the client will automatically attempt to download this dataset from the remote instance. Only one result can be returned at a time, and if you ever need to download more, simply submit a new workflow with only the return statement.
As an alternative to using the generic function, the
visualization
package exposes its individual plot generation logic as separate functions. It might be a fun exercise to try and add these yourself, by usingbrane inspect
and the package's code itself.
And that's it! You can now save and close your workflow file(s), and then move on to the next step: executing it.
Local execution
We can execute the workflow locally first to see if it all works properly. To do so, open up a terminal, and then run the following:
brane run <PATH_TO_WORKFLOW>
If your workflow works, you should see it blocking which indicates it is working. Eventually, the workflow should return and show you where it stored the final result of the workflow. If not, then it will likely show you an error of what went wrong, which may be anything from passing the wrong arguments to forgetting a semicolon (the latter tends to generate "end-of-file" errors, as do missing parenthesis errors).
Tip: If you want to better monitor the progression, insert
println()
calls in your workflow! It takes a single argument, which will always be serialized to a string before printing it to stdout. By mixingprint()
(print without newline) andprintln()
, you can even write formatted strings.
After having added some additional println()
statements, you might see something like the following:

(You can ignore the warning message)
You can then inspect the index file by navigating to the suggested folder, and then opening index.html
. If you have a browser like Firefox installed, you can also run:
firefox "<PATH>/index.html"
to open it immediately, where you can replace <PATH>
with the path returned by BRANE.
You will then see a nice web page containing the plots generated about the model and the dataset. It should look something like:
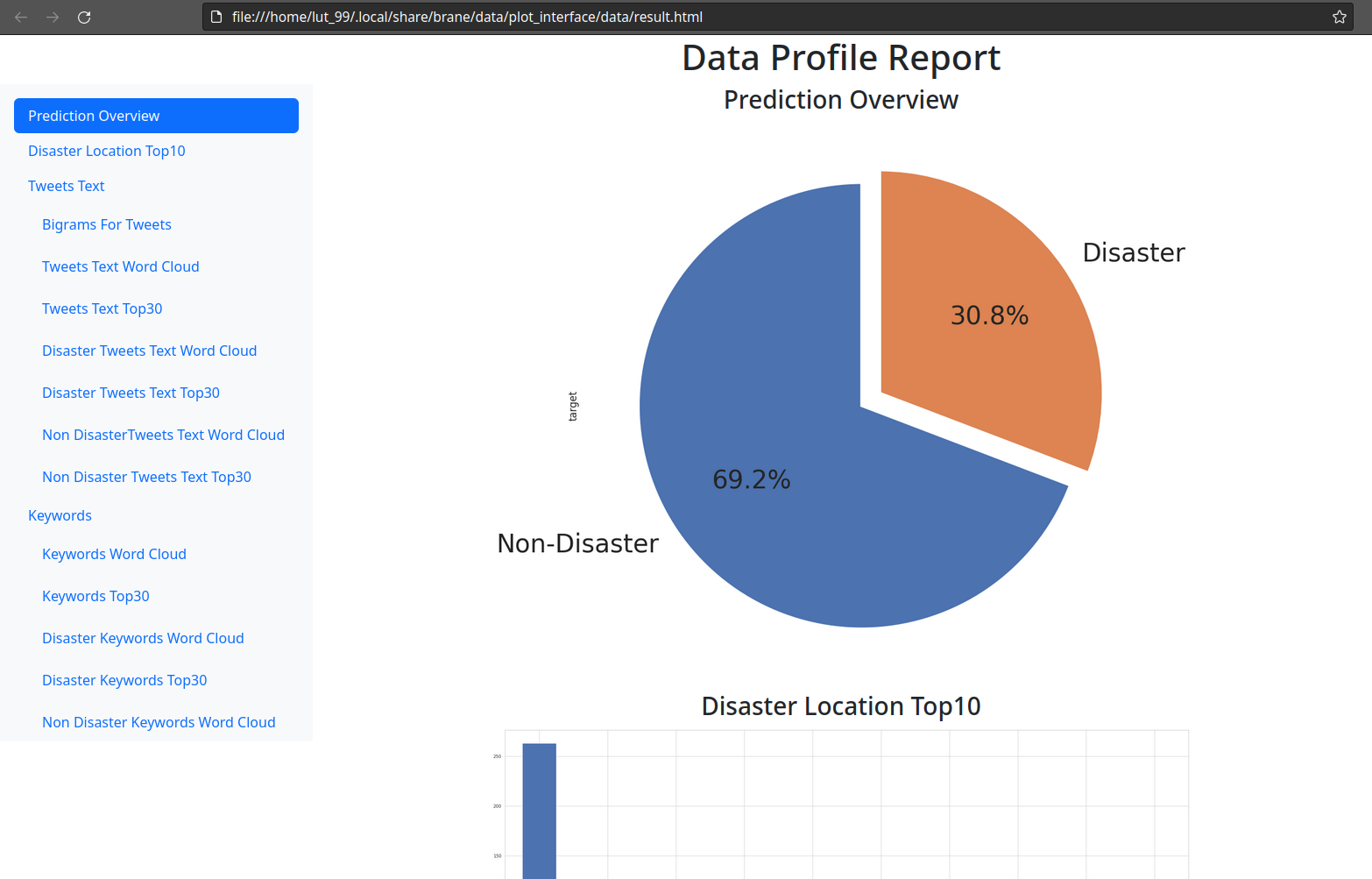
Remote execution
More interesting than running the code locally, though, would be to run it remotely on a running BRANE instance.
For the purpose of the tutorial, we have setup an instance with two worker nodes: one resides at the University of Amsterdam (at the OpenLab cluster), and the other resides at SURF's ResearchCloud environment.
Updating the workflow
We can run the workflow on either of these locations. To do so, you have to do a small edit to your workflow, because the planner of the framework is a little too simplistic in its current form. Open your workflow.bs
file again, and wrap the code in the following:
on "uva" {
// ... your code
}
(You can leave the import
statements outside of it, if you like)
This on-struct tells the framework that any task executed within must be run on the given location. Because there are two locations, you can use two different identifiers: uva
, for the University of Amsterdam server, or surf
for the SURF server.
The reason that you have to manually specify this is because both sites have access to the required dataset. This means that the framework has to equally possible locations, and to avoid complications with policy, the framework just gives up and requires the programmer to manually make the decision where to run it. In the future, this should obviously be resolved by the framework itself.
You can now close your file again, after having saved it.
Adding the instance to your client
Then, go back to your terminal so that we can register this instance with your client.
You can register an instance to your client by running the following command:
brane instance add brane01.lab.uvalight.net --name tutorial --use
This will register an instance who's central node is running at brane01.lab.uvalight.net
. The ports are all default, so there is no need to specify them. The tutorial
-part is just a name so you can recognize it later, so you can replace it with something else if you want.
If the command runs successfully, you should see something like:

You can then query the status of the instance by running:
brane instance list --show-status

We now know the instance has been added successfully!
Adding certificates
Before we can run the workflow in the remote environment, we have to add a certificate to our client so that the remote domains know who they might be sharing data with. In typical situations, this requires contacting the domain's administrator and asking them for a certificate. However, because this is a tutorial, you will all be working as the same user.
Note that you will need new certificates for every domain, since you may not be involved with every domain. Thus, you can download the certificates for the University of Amsterdam here, and the certificates for the SURF server here.
Obviously, posting your private client key on a publicly available website is about the worst thing you can do, security-wise. Luckily for us, this tutorial is about the BRANE framework and not security best practises - but just be aware this isn't one.
Download both of these files, and extract the archives. Then, for each of the two directories with certificates, run the following command to add them to the client:
# For the University of Amsterdam
brane certs add ./ca.pem ./client-id.pem --domain uva
# For SURF
brane certs add ./ca.pem ./client-id.pem --domain surf
Unfortunately, there is a problem with certificate generation that does not properly annotate the domains in the certificates which causes the warnings to appear. However, this is not an issue, since the certificates are still signed with the private keys of the domains, and thus still provide reliable authentication.
After they are added, you can verify they exist with:
brane certs list

You are now ready to run your workflow online!
The final step
With an instance and certificates setup, and the proper instance selected, you can then run your workflow on the target instance. Normally, you would have to push your package to the instance (brane push <package name>
) and make sure that the required datasets are available (this can only be done by the domain's administrators). However, for the tutorial, both of these steps have already been done a-priori.
Thus, all is left for you to execute your workflow remotely. Do so by running:
brane run <PATH_TO_WORKFLOW> --remote
You might note this is exactly the same command as to run it locally, save for the additional --remote
flag. Subsequently, your output should also be roughly the same:
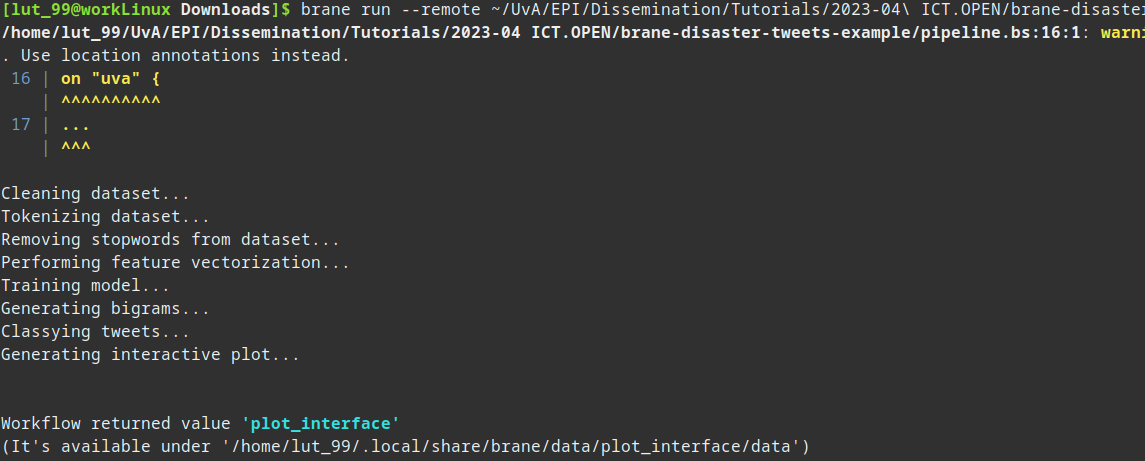
In the final step, the part with Workflow returned value...
, the dataset is downloaded to your local machine. This means it is available in a similar manner as for local datasets, except it has now been executed remotely.
If you use the
--debug
flag, you might see that the final result is actually downloaded from a different location than where you executed the workflow. This is because the resulting dataset is available on both sites (under the same identifier), and because the on-struct only affects tasks, not the builtincommit_result
-function. Whether this has to be changed in the future remains to be seen, but just repeat the execution of your workflow a few times to also see the download from the other location.
Conclusion
Congratulations! You have now written a more complex workflow for a more complex package, and successfully ran it online. Hopefully, you find the framework (relatively) easy to work with, and enjoyed the experience of getting to know it!
If you still have time left, take the opportunity to play around and ask questions. You can select various topics in the sidebar to the left of this wiki page to find more explanations about the framework. Especially the topics on BraneScript might be interesting, to learn about more possibilities that can be done with the workflow language.
Note, however, that the wiki is still incomplete and unpolished, like the framework itself. If you want to know anything, however, feel free to ask it to the tutorial hosts!
Thanks for attending :)
Brane Demo at the UMC Utrecht
The second tutorial given about Brane was held at the UMC Utrecht to conclude a Proof-of-Concept performed with them, which focused on Brane serving as data sharing infrastructure for various analysis on pseudonamised patient data.
The tutorial is written for framework version 3.0.0.
The demo is split in two halves: the first half consists of a presentation introducing the framework at a generic SIG-meeting, whereas the second half features a hands-on session and a more technical presentation about the setup of Brane in the Proof-of-Concept.
The following resources are used, which are hosted on this website:
- First half: SIG-meeting
- Slides (here)
- Second half: Workshop
Part 1: Hello, world!
In this document, we detail the steps to take to write a simple package within the EPI Framework (also called the Brane Framework). Specifically, this tutorial will focus on how to install the CLI client (the brane
executable), build a hello_world
package and then call the hello_world()
function within that package on a local machine. Finally, we will also practise submitting the code to a remote machine.
Background
The framework revolves around workflows, which are high-level descriptions of an algorithm or data processing pipeline that the system will execute. Specifically, every workflow contains zero or more tasks, which are conceptual functions that take an in- and output, composed in a particular order or control flow. It helps to think of them as graphs, where the nodes are tasks that must be called, and the edges are some form of data flowing between them.
We could formalise a particular data pipeline as a workflow. For example, suppose we have the following function calls:
g(f(f(input)), h(f(input)))
We can then represent this as a workflow graph of tasks that indicates which tasks to execute and how the data flows between them. This is visualised in Figure 1.
Figure 1: A very simple workflow using three tasks, f
, g
and h
. The nodes represent a function call, whereas the edges represent some data dependency. Specifically, this workflow depicts f
has to be run first, then a second call of f
and a new call of h
can run in parallel because they don't depend on each other, after which a third function g
must be called.
While workflows can be expressed in any kind of language, the EPI Framework features its own Domain-Specific Language (DSL) to do so, called BraneScript1. This language is very script-like, which allows us to think of tasks as a special kind of function. Any control flow (i.e., dependencies between tasks) is then given using variables and commonly used structures, such as if-statements, for-loops, while-loops, and less commonly used structures, such as on-structs or parallel-statements.
There is a second DSL, Bakery, which is more unique to the framework and features a natural language-like syntax. However, this language is still in development, and so we focus on BraneScript.
Objective
In this tutorial, we will mainly focus on creating a single task to execute. Traditionally, this task will be called hello_world()
, and will return a string "Hello, world!"
once it is called. To illustrate, the following Python snippet implements the logic of the task:
def hello_world():
return "Hello, world!"
By using print(hello_world())
, we can print Hello, world!
to the terminal. In the EPI Framework, we will implement the function as a task, and then write a very simple workflow that implements the print-part.
Installation
To start, first download the brane
executable from the repository. This is a command line-based client for the framework, providing a wide range of tools to use to develop packages and workflows for the EPI Framework. We will use it to build and then test a package, which can contain one or more tasks. Since we are only creating the hello_world()
task, our package (called hello_world
) will contain only one task.
The executable is pre-compiled for Windows, macOS (Intel and M1/M2) and Linux. The binaries in the repository follow the pattern of <name>-<os>-<arch>
, where <name>
is the name of the executable (brane
for us), <os>
is an identifier representing the target OS (windows
for Windows, darwin
for macOS and linux
for Linux), and <arch>
is the target processor architecture (x86_64
, typically, or aarch64
for M1/M2 Macs).
To make your life easy, however, you can directly download the binaries here:
When in doubt, choose
x86_64
for your processor architecture. Or ask a tutorial host.
Once downloaded, it is recommended to rename the executable to brane
to follow the calling convention we are using in the remainder of this document. Open a terminal in the folder where you downloaded the executable (probably Downloads
), and run:
:: For Windows
move .\brane-windows-x86_64 .\brane
# For macOS (Intel)
mv ./brane-darwin-x86_64 ./brane
# For macOS (M1/M2)
mv ./brane-darwin-aarch64 ./brane
# For Linux
mv ./brane-linux-x86_64 ./brane
If you are on Unix (macOS/Linux), you probably want to execute a second step: by just renaming the executable, you would have to call it using ./brane
instead of brane
. To fix that, add the executable to somewhere in your PATH, e.g.:
sudo mv ./brane /usr/local/bin/brane
If you installed it successfully, you can then run:
brane --version
without getting not found
-errors.
If you don't want to put
brane
in your PATH, you can also replace all occurrences ofbrane
with./brane
in the subsequent commands (or any other path/name). Additionally, you can also run:export PATH="$PATH:$(pwd)"
to add your current directory to the PATH variable. Note that this lasts only for your current terminal window; if you open a new one or restart the current one, you have to run the
export
-command again.
Writing the code
The next step is to write the code that we will be running when we execute the task. In the EPI Framework, tasks are bundled in packages; and every package is implemented in a container. This means that every task has its own dependencies shipped within, and that multiple tasks can share the same dependencies. This also means that a task can be implemented in any language, as long as the program follows a particular convention as to how it reads input and writes output. Specifically, the EPI Framework will call a specific executable file with environment variables as input, and then retrieve return values from it by reading the executable's stdout
.
For the purpose of this tutorial, though, will choose Python to implement our hello_world
-function. Because our function is so simple, we will only need a single file, which we will call hello.py
. Create it, and then write the following in it (including comments):
#!/usr/bin/env python3
def hello_world():
return "Hello, world!"
print(f'output: "{hello_world()}"')
Let's break this down:
- The first line,
#!/usr/bin/env python3
is a line that tells the operating system that this file is a Python script (it defines it must be called with thepython3
executable). Any file that has this on the first line can be called by just calling the file instead of having to prefixpython3
, e.g.,
instead of./hello.py
This is important, because the framework will use the first calling convention.python3 hello.py
- The function,
def hello_world(): ...
, is the same function as presented before; it simply returns the"Hello, world!"
string. This is actually the functionality we want to implement. - The final line,
print(f'output: "{hello_world()}"')
prints the generated string to stdout. Note, however, that we wrap the value in quotes ("
) and prefix it withoutput:
we do this because of the convention that packages for the EPI Framework have to follow. The framework expects the output to be given in the YAML format, under a specific name. We chooseoutput
(see below).
And that's it! You can save and close the file, while we will move to the second part of a package: the container.yml
file.
Writing the container.yml
A few text files do not make a package. In addition to the raw code, the EPI Framework also needs to know some metadata of a package. This includes things such as its name, its version and its owners, but, more importantly, also which tasks the package contributes.
This information is defined using a file conventionally called container.yml
. This is another YAML file where the toplevel keys contribute various pieces of metadata. Create a file with that name, and then write the following to it:
# A few generic properties of the file
name: hello_world
version: 1.0.0
kind: ecu
# Defines things we need to install
dependencies:
- python3
# Specifies the files we need to put in this package
files:
- hello.py
# Defines which of the files is the file that the framework will call
entrypoint:
kind: task
exec: hello.py
# Defines the tasks in this package
actions:
'hello_world':
command:
input:
output:
- name: output
type: string
This is quite a lot, so we will break it down in the following subsections. Every subsection will contain the highlighted part of the container.yml
first, and then uses three dots (...
) to indicate parts that have been left out for that snippet.
Minimal metadata
# A few generic properties of the file
name: hello_world
version: 1.0.0
kind: ecu
...
The top of the file starts by providing the bare minimum information that the EPI Framework has to know. First are the name of the package (name
) and the version number (version
). Together, they form the identifier of the package, which is how the system knows which package we are calling tasks from.
Then there is also the kind
-field, which determines what kind of package this is. Currently, the only fully implemented package kind is an Executable Code Unit (ECU), which is a collection of arbitrary code files. However, other packages types may be supported in the future; for example, support for external Workflow files (BraneScript/Bakery) or, if network support is added, OpenAPI containers.
Specifying dependencies
...
# Defines things we need to install
dependencies:
- python3
...
Because packages are implemented as containers, we have the freedom to specify the set of dependencies to install in the container. By default, the framework uses Ubuntu 20.04
as its base image, and the dependencies specified are apt-packages. Note that the base container is fairly minimal, and so we have to specify we need Python installed (which is distributed as the python3
-package).
Collecting files
...
# Specifies the files we need to put in this package
files:
- hello.py
...
Then the framework also has to know which files to put in the package. Because we have only one file, this is relatively simply: just the hello.py
file. Note that any filepath is, by default, relative to the container.yml
file itself; so by just writing hello.py
we mean that the framework needs to include a file with that name in the same folder as container.yml
.
The files included will, by default, mimic the file structure that is defined. So if you include a file that is in some directory, then it will also be in that directory in the resulting package. For example, if you include:
files: - foo/hello.py
then it will be put in a
foo
directory in the container as well.
Setting the entrypoint
...
# Defines which of the files is the file that the framework will call
entrypoint:
kind: task
exec: hello.py
...
Large projects typically have multiple files, and only one of them serves as the entrypoint for that project. Moreover, not every file included will be executable code; and thus it is relevant for the framework to know which file it must call. This is specified in this snippet: we define that the hello.py
file in the container's root is the one to call first.
As already mentioned, the framework will call the executably "directly" (e.g., ./hello.py
in this case). This means that, if the file is a script (like ours), we need a shebang (e.g., #!/usr/bin/env python3
) string to tell the OS how to call it.
Even if your package implements multiple tasks, it can only have a single entrypoint. To this end, most packages define a simple entrypoint script that takes the input arguments and uses that to call an appropriate second script or executable for the task at hand.
Defining tasks
...
# Defines the tasks in this package
actions:
hello_world:
command:
input:
output:
- name: output
type: string
The final part of the YAML-file specifies the most important part: which tasks can be found in your container, and how the framework can call them.
In our container, we only have a single task (hello_world
), and so we only have one entry. Then, if required, we can define a command-line argument to pass to the entrypoint to distinguish between tasks (the command
-field). In our case, this is not necessary because we only have a single one, and so it is empty.
Next, one can specify inputs to the specific task. These are like function arguments, and are defined by a name and a specific data type. At runtime, the framework will serialize the value to JSON and make these available to the entrypoint using environment variables. However, because our hello_world()
function does not need any, we can leave the input
-field empty too.
Finally, in the output
section, we can define any return value our task has. Similar to the input, it is defined by a name
and a type
. The name given must match the name returned by the executable. Specifically, we returned output: ...
in our Python script, meaning that we must name the output variable output
here as well. Then, because the output itself is a string, we denote it as such by using the type: string
.
In summary, the above actions
field defines a single function that has the following pseudo-signature:
hello_world() -> string
Building a package
After you have a container.yml
file and the matching code (hello.py
), it is time to build the package. We will use the brane
CLI-tool for this, and requires Docker and the Docker Buildx-plugin to be installed.
On Windows and macOS, you should install Docker Desktop, which already includes the Buildx-plugin. On Linux, install the Docker engine for your distro (Debian, Ubuntu, Arch Linux), and then install the Buildx plugin using:
# Install the executable
docker buildx bake "https://github.com/docker/buildx.git"
mkdir -p ~/.docker/cli-plugins
mv ./bin/build/buildx ~/.docker/cli-plugins/docker-buildx
# Create a build container to use
docker buildx create --use
If you have everything installed, you can then build the package container using:
brane build ./container.yml
The executable will work for a bit, and should eventually let you know it's done with:

If you then run
brane list
you should see your hello_world
container there. Congratulations!
Running your package locally
All that remains is to see it in action! The brane
executable has multiple ways of running packages locally: running tasks in isolation in a test-environment, or by running a local workflow. We will do both of these in this section.
The test environment
The brane test
-subcommand implements a suite for testing single tasks in a package, in isolation. If you run it for a specific package, you can use a simple terminal interface to select the task to run, define its input and witness its output. In our case, we can call it with:
brane test hello_world
This should show you something like:

If you hit Enter, the tool will query you for input parameters - but since there are none, instead it will proceed to execution immediately. If you wait a bit, you will eventually see:

And that's indeed the string we want to see!
The first time you run a newly built package, you will likely see some additional delay when executing it. This is because the Docker backend has to load the container first. However, if you re-run the same task, you should see a significant speedup compared to the first time because the container has been cached.
Running a workflow
The above is, however, not very interesting. We can verify the function works, but we cannot do anything with its result.
Instead of using the test environment, we can also write a very simple workflow with only one task. To do so, create a new file called workflow.bs
, and write the following in it:
import hello_world;
println(hello_world());
Let's examine what happens in this workflow:
- In the first line,
import hello_world;
, we tell the framework which package to use. We refer our package by its name, and because we omit a specific version, we let the framework pick the latest version for us (we could have usedimport hello_world[1.0.0];
instead). - In the second line,
println(hello_world());
, we call ourhello_world()
task. The result of it will be passed to a builtin function,println()
, which will print it to the stdout.
Save the file, close the editor, and then run the following in your terminal to run the workflow:
brane run ./workflow.bs
If everything is alright, you should see:

The
brane
-tool also features an interactive Read-Eval-Print Loop (REPL) that you can use to write workflows as well. Runbrane repl
, and then you can write the two lines of your workflow separately:![]()
Because it is interactive, you can be more flexible and call it repeatedly, for example:
![]()
Simply type
exit
to quit the REPL.
Running your package remotely
Of course, running your package locally is good for testing and for tutorials, but the real use-case of the framework is running your code remotely on a Brane instance (i.e., server).
Adding the instance
First, we have to make the brane
-tool aware where the remote Brane instance can be found. We can use the brane instance
-command for that, which offers keychain-like functionality for multiple instances to easily switch between.
Prior to this tutorial, we've setup an instance at brane01.lab.uva.light.net
. To add it to your client, run the following command:
brane instance add brane01.lab.uvalight.net -a 50051 -d 50053 -n demo -u
To break down what this command does:
brane instance add brane01.lab.uvalight.net
tells the client that a new instance is being defined that is found at the given host;-a 50051
tells the client that the API service is found at port 50051 (the central registry service);-d 50053
tells the client that the driver service is found at port 50053 (the central workflow execution service);-n demo
tells the client to call this new instancedemo
, which is an arbitrary name only useful to distinguish multiple instances (you can freely change it, as long as it's unique); and-u
tells the client to use the instance as the new default instance.
Once the command completes, you can run the following command to verify it was a success:
brane instance list

Pushing your package
Now that you defined the instance to use, we can push your package code to the server so that it may use it.
This is done by running the following command:
brane push hello_world
This will push the specified package hello_world
to the instance that is currently active. Wait for the command complete, and once it has, we can prepare the workflow itself for remote execution.
Note that this instance is shared by all participants in the system; so if you just upload your own package with the
hello_world
-name, you will probably overwrite someone else's. To avoid this problem, re-build your package with a unique name before pushing.
Adapting the workflow
In the ideal case, Brane can take a workflow that runs locally and deduce by itself where the steps in the workflow must be executed (i.e., plan it). However, unfortunately, the current implementation can't do this (ask me why :) ), and so we have to adapt our workflow a little bit to make it compatible with the instance that we're going to be running on.
Our instance has two nodes: worker1
and worker2
. To tell Brane which of the instance we want to use, we can wrap the line that has the hello_world()
-call in a so-called on-struct to force Brane to run it on that node.
Open the workflow.bs
-file again, and write:
import hello_world;
on "worker1" {
println(hello_world());
}
Save it and close it, and we're ready to run your workflow remotely!
Running remotely
In the first step of running the workflow remotely, we already defined the instance and marked it as default; so all that needs to be done is to run the same command again as before to execute the workflow, but now with the --remote
-flag to indicate it must be executed on the currently active instance instead:
brane run ./workflow.bs --remote

And that's it! While it looks like there isn't a lot of difference, your code just got executed on a remote server!
You may see warnings relating to the 'On'-structures being deprecated (see the image above). This can safely be ignored; they will be replaced by a better method soon, but this method is not implemented yet.
Using the IDE
If the workflow is going to be remotely, one can also step away from the CLI-tool and instead use the Brane IDE-project, which is built on top of Jupyter Lab to provide a BraneScript notebook interface.
Note that currently, only writing and running workflows is supported (i.e., the
brane run ...
command). Managing packages still has to be done through the CLI.
To use it, download the source code from the repository and unpack it. Also download the Brane CLI library, which is used by the IDE to send commands to the Brane server. You can download it here. Unpack it as well, and place the libbrane_cli.so
file in the root folder of the Brane IDE repository.
Once you have everything in place, you can launch an IDE connecting to this tutorial's Brane instance by running in the repository root:
./make.py start-ide -1 http://brane01.lab.uvalight.net:50051 -2 grpc://brane01.lab.uvalight.net:50053
The command may take a second to complete, because it will first build the container that will run the server.


Once done, you can copy/paste the suggested link to your browser, and you should be greeted by something like:

If you click on the BraneScript
-tile, you should see a notebook; and now you can run BraneScript workflows in the classic notebook fashion!

Conclusion
And that's it! You've successfully written your first EPI Framework package, and then you ran it locally and verified it worked.
In the second half of the tutorial, we will focus more on workflows, and write one for an extensive package already developed by students. You can find the matching handout here.